Multiple hypothesis tracking
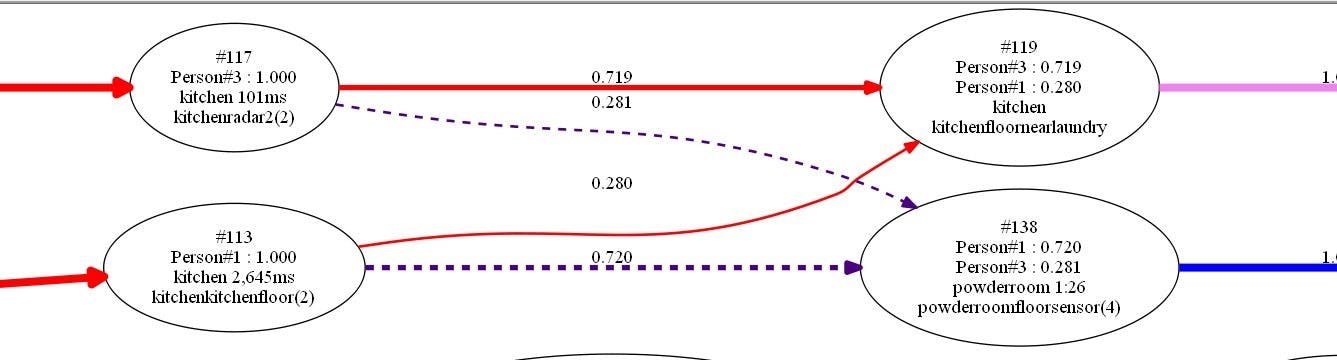
My home automation system collects a lot of data from the 250+ sensors in it. These sensors feed my complex state machine logic to decide which rooms are occupied and which lights to put on or turn off. It works well, but it's not perfect so it errs on the side of leaving lights on longer than it needs to in rooms that you've passed through. It also doesn't learn your habits, it just has some tried and tested state machine transitions that work really well for most situations. So for many years I've been considering what kind of machine learning I could apply in order to say for certain which rooms are occupied and which are not and how many people are in each room.
This is a very hard problem for ML because it isn't just a sequence, is temporal data: it's not just the sequence [A-B-C] that matters but the time between each of those events and
those intervals are hard to classify. In some cases we care about triggers happening seconds apart, in some minutes apart, in some ~24 hours apart, a week apart
or even a year apart. And in most cases those intervals aren't precise, some days you get up early, some days you don't. So good luck just throwing that at an RNN and
hoping that it will spot the birthday celebration state
that happens once a year for each person in the house.
It's also a hard problem because the data is fairly sparse. A front door for example might be opened just a couple of times a week. So although I have 150GB+ of historical data for my system there are still fairly few instances of some events like 'holiday party' to analyze.
But the hardest problem is that none of this data is labeled: nobody has annotated every event and state transition with a label that says "4 people in home, 1 in kitchen, 1 in study and 2 in living room" which might allow a machine learning algorithm to be trained, and I'm certainly not going to sit here for a month capturing a label for each transition even if the house could chat to me and ask "what state are we in now" which I guess it could.
And so, after years of research and reading papers on machine learning to find a solution, I kept ending back on the research around Multiple Hypothesis Tracking. I began prototyping my own version many years ago but finally, driven by the lack of labeled data for machine learning, I went ahead and finished it. And it works!
In essence MHT works by maintaining a list of hypotheses, each of which has an associated probability and a set of numbers saying how many people it thinks are in each room. Whenever it receives a sensor event in a given room it updates this list using probabilities for the possible transitions that this sensor event could imply.
For home automation these transitions are (in order from most likely to least likely):
- Someone already in this room just moved again
- Someone moved from a neighboring room to this room
- Someone jumped from a room elsewhere to this room bypassing some other rooms where sensors might not have tripped
- Someone spontaneously appeared in this room (Star Trek transporter!) because they arrived from somewhere outside the system, or we had failed to track them earlier, or the system had just restarted
Fortunately my home automation system treats the house as a graph so it understands which rooms are neighbors and even how many steps any given room is from all the other rooms in the house.
// Get a sorted list of rooms by distance
// (a tuple of room and distance from the first room)
var roomsByDistance = room.RoomsByDistance
On a regular interval it also recalculates all the probabilities assuming that in any room where no motion has been detected there is now a reduced probability that there is someone in that room. Eventually after a long time that room is marked not-occupied but typically it drops off the list much sooner as the system detected somone moving from it to another room.
Of course, after just a few iterations this list of possible scenarios gets impossibly long. Each sensor event spins off three or more new hypotheses. So, as is typical in this method, my algorithm trims the list of hypotheses removing all the unlikely ones. For example, on testing it thought, after just a few minutes that there might be four people in the bedroom but the associated probability of 10⁻¹⁵ corresponded roughly to the likelihood of an alien invasion starting in the bedroom so that hypothesis was trimmed.
Here's a worked example, starting from the empty set, or null hypothesis where the house is certain that nobody is home and triggering a single sensor repeatedly.
START
p(1) {}
ONE MOVE
p(0.81) {Office:1}
p(0.19) {}
After the first motion detection it is fairly certain that there is one person in the office (81% chance) and gives a fairly low probablity for the house being empty (19%) which reflects that this could just be a false alarm.
SECOND MOVE
p(0.9638271) {Office:1}
p(0.0361) {}
p(7.29E-05) {Office:2}
After the second motion detection it becomes even more certain that there is someone in the office, but also thinks that there could be two people there (the space alien materialized scenario, or maybe there really were two people there and we just didn' notice). The aggregate probability of the office being occupied is now up to 0.9639.
THIRD MOVE
p(0.992981355561) {Office:1}
p(0.006859) {}
p(0.000159637878) {Office:2}
p(6.561E-09) {Office:3}
After the third motion event it's now very certain that there is one person in the office (0.99298) and even more certain that there is one or more people in the office (sum = 0.993141) and very certain that the house is not empty.
Status
After just one day I'm fairly confident that this approach is working well and that it's going to be much better than my old Occupied=true/false approach which required absolute certainty that a room was empty for a very long time before shutting lights off there. If you are home alone now you can walk around and the probability that you are in a given room is very high and the probability that you are somewhere else is very low. As you follow a path through the house you reinforce your position and the probability goes up even though you are moving rooms.
Next steps
Moving from true/false to probabilistic values for occupancy (and maybe other features in the house) is going to bring a huge change in the way my system works. Lights will be able to turn off much more rapidly in rooms that are no longer occupied as the system will have tracked you from one room to another.
But it also raises lots of new issues:
Q: How certain do you need to be to turn a light on?
A: not very certain, just do it (except in bedroom where the opposite holds!).
Q: How certain do you need to be to turn a light off?
A: much more certain, you don't want to put people in the dark
Closing thoughts
The application of this approach to home automation could have many interesting business aspects to it also. I will be able to tell you the probability of each room being occupied on each day of the week and the overall chance that the house is occupied for any hour of the day.
- What if your insurance premium was calculated on that basis?
- Could that letter from the energy company that says I'm using too much electricity factor in the fact that I work from home and realize that actually I'm actually in one of the least energy consuming homes (per occupied hour) in the neighborhood?
- Could an alarm system based on this give the operator (and you) a probability with each alarm call
19% chance there's an intruder in the basement
which might save a lot of false alarms and needless call out for the police?